By Cynthia Challener, CoatingsTech Contributing Writer
Digitalization is occurring across all manufacturing industries, and the coatings sector is no exception. The quantity of data that can be leveraged to improve all business activities—from new product development to production to customer service—is increasing dramatically. The challenge is to determine where and how to apply technologies such as artificial intelligence (AI), machine learning (ML), and natural language processing (NLP) and how to make the data on hand relevant to the problem or question of interest. These questions and others were considered by members of the coatings value chain and their insights are presented below.
Participants in the Discussion Included:
Richie Ramsden, head of Data Science at AkzoNobel’s Innovation Incubator;
Aleta Richards, senior vice president of Sales & Market Development for the Coatings, Adhesives & Specialties business of Covestro in North America;
Jeff Millman, senior engineering manager; Heather Kettelhohn, supply chain director; Michael Devon, intellectual property director; Guillaume Deudon, customer experience services; Sarah Eckersley, R&D director; Jan Weernink, marketing director; and Sharon Kraus, TS&D director, Dow Coating Materials;
Henrik Hahn, chief digital officer (CDO) of Evonik Industries and chairman of the Management Board of Evonik Digital GmbH;
Steve Risser, senior research leader, and Jared Schuetter, senior data scientist, Battelle;
Erik Sapper, assistant professor in the Western Coatings Technology Center and the Dept. of Chemistry and Biochemistry at California Polytechnic State University
What types of Big Data can be leveraged by the coatings industry to facilitate research, development, and innovation in general?
Sapper, Cal Poly: We need to be asking three questions when it comes to data needs in our industry. What data do we have? What data do we need? And what questions are we trying to answer? A lot of valuable data already exists, but it is tied up in reports, published literature, or subject matter expertise. The data is there, but not collected in a way that allows helpful artificial intelligence and machine learning projects to be performed. Understanding what type of data is needed for a particular project is the first step in identifying where that data might already exist. If it doesn’t exist, that’s when experimentation can help by filling in the gaps in the available data.
Every imaginable data type is amenable to artificial intelligence and machine learning implementations. In the coatings industry, the most helpful data is likely going to include small molecule structure-property data, polymer synthesis procedures and outcomes, formulation recipes, formulation design space data, “time zero” performance data that is measured after synthesis, formulation, or product application, and—especially—service life data, so that predictions can be made about the useful longevity of the product. All of this is largely quantitative and structured data found in tables and spreadsheets. Unstructured data in the form of textual internal reports, literature, technical data sheets, and even online data serve as a vast additional source of possibly useful data. Here, the challenge lies in creating natural language processing tools so that algorithms can “read” these technical documents and extract useful information like a human.
Ramsden, AkzoNobel: Holistic digital transformation of any well-established industry requires progress in three areas:
- Customer Experience: creating a customer experience that keeps customers coming back. Creating new ways to engage customers, delivering desirable products before customers know they want it.
- Manufacturing Excellence: Using data to further embed the principles of Lean manufacturing. Predictive maintenance reduces downtime—and optimizes manufacturing schedules.
- Disruption: Often overlooked in digital transformation, creating new transformative business models from Big Data. Migrating business models to service-lead, rather than transactional, but also creating new markets entirely.
A good example of a Disruption project is our Marine Fouling Challenge database. Created to support our coatings specification tools (like Intertrac and Intertrac Vision), it has value by providing Global Fouling Challenge maps to new customers, for example, in carrying out risk analysis assessments or providing up-to-date status reporting.
Sensors are likely to facilitate a lot of digital transformation, especially in the manufacturing sphere. Internet of things (IoT) sensors create the possibility of digital twins of manufacturing sites, which allow decisions to be made in real-time to further embed Lean manufacturing.
Devon, Dow Coating Materials: At Dow Coating Materials, examining large Intellectual Property (IP) data sets along with advanced analytics on Market Research-related data is used to uncover many different types of information. Specifically, hidden patterns, unknown correlations, market trends, and customer preferences can help the business make informed decisions regarding where it can participate and whether it should participate within a given segment. Coating related IP data is well suited for the deep insights that big data techniques can provide because it is global, high varied, and continually changing. Applying the results of the Big Data analysis is also used to derive the best business strategy for a given market segment.
Richards, Covestro: Covestro is leveraging data related to decades of information . . . laboratory results, testing, customer trends, orders, and projects we’ve completed for customers. All this information is in a data pool that is available to us. There is a very high volume of information, and we are determining how best to utilize this wealth of data to help inform our growth strategy, our sustainability efforts, and so much more.
Hahn, Evonik Industries: In the end, it is all about Information that acknowledges customer needs. What is new is the inclusion of unstructured data and the smart combination with existing application-related structured data including those from high throughput experimentation. This new approach will, for example, allow derivation of formulation suggestions based on machine learning rather than solely from experiment. So, leveraging data will ultimately help to shorten development times.
What are other applications for Big Data in the coatings industry besides new product development?
Hahn, Evonik Industries: First of all, data thinking in the coatings industry should not be limited to Big Data. The intelligent use of data is more a matter of Smart Data. Potential use cases can be found for any business process—from the utilization of the information flow within the supply chain network to improving the accuracy of sales forecasts enabling effective production planning.
Ramsden, AkzoNobel: Potential applications for Big Data are wide-ranging in our industry— from in-field performance predictions to coatings that tell the asset owners when they need attention.
Big Data allows us to answer many questions that, up to this point, have been impossible to address. Data frequency, as well as the range of data sources, allows our industry to measure, track, and affect many more elements in our manufacturing, customer experience, and new markets than ever before.
The crucial applications for Big Data will come from application of existing knowledge and thinking, including innovation, using data as a tool. Data in itself has no value, but using the data to gain insight, or to drive a change for the better, is where Big Data, AI, and ML will have the largest impact.
There are already tools built on Big Data and AI in our industry—for example, International Paint’s Intertrac Vision cost-benefit-analysis tool, which provides a full overview of the impact of marine vessel coating decisions to ensure that coating customers can make fully informed decisions.
It is crucial that the problems we tackle with data are real, and that data is viewed as a tool to help us design solutions to satisfy them.
Richards, Covestro: Digitalization makes it easier and faster to collaborate within our company, all along the value chain and, of course, with our customers. Speaking more specifically about our customers, one of our top objectives related to digitalization is enhancing the customer experience. This summer we launched our online digital solutions center, which is a central place our customers can visit to find case studies, hundreds of pages of regulatory data, and other information. The amount of information is exhaustive. While much of it was available before, we focused on packaging it so that it can be used more effectively. Data overload is something we can all relate to in our personal and professional lives; the trick is finding the data that is most meaningful for you.
At Covestro, we’ve also developed a digital tool to help our customers streamline product selection. Using this tool, customers select the properties they are looking for. Based on these inputs, we can efficiently determine the optimal formulation for achieving it. The tool is very flexible, allowing customers to pull in their own data to find a very specific solution.
Deudon, Dow Coating Materials: Dow has developed collection, organization, analysis, and visualization methodologies that unlock insights from business-relevant internal and external text sources. While Dow does not sell directly to consumers, we have found it valuable to mine social media to gauge consumer sentiment on our customers’ products. Armed with those insights, Dow has been able to suggest alternative approaches and improvements to its customers that benefit the end consumer.
Risser, Battelle: Leveraging Big Data in combination with machine learning has the potential to find hidden relationships that normally would not be sought and haven’t really been studied before. This type of information can be used to improve many different business activities, including innovation efforts. For instance, it may be used to explore product failures—to tease out relationships or effects that aren’t yet understood. In the process environment, leveraging trending data can aid in optimization of process parameters and enhanced process control.
Schuetter, Battelle: Aging of the workforce in the coatings and many other industries could potentially lead to loss of institutional knowledge and even to some extent the capability to maintain product development streams. While coating formulation development is in many ways still more of an art form than a science, the use of Big Data and artificial intelligence may be able to help address this issue. For instance, the Defense Advanced Research Projects Agency (DARPA) is funding the development of adaptive AI models that operate over ontologies that capture knowledge in the form of relationships between different concepts and measurable outcomes. These systems are intended to make decisions on the fly and reproduce the types of decisions a person with a lot of knowledge and experience would make. A new release on the project can be found at https://www.darpa.mil/news-events/2018-09-07.
Sapper, CalPoly: Service life prediction methods may be improved with the development of large data sets of coating performance over time, especially if driven by sensors and IoT collection methods.
Supply chain optimization, SKU management, and inventory planning may be improved when cost data, production timelines, and logistics information is coupled with existing scientific domain knowledge. For example, a project might consider where products in a portfolio overlap in different areas of performance, indicating the opportunity for product removal. The tool or set of models may help identify areas in a portfolio where no product currently exists.
Text mining and natural language processing is being used to uncover the popular sentiment around current chemical trends, buzzwords, and materials of concern. This is the focus of the current ACA Big Data effort, which crawls the web and updates a user dashboard with perceived risk and chatter—or the volume of online text or mentions—around particular materials of concern.
How can AI and ML benefit members of the coatings value chain?
Sapper, CalPoly: Artificial intelligence and machine learning implementations are providing accelerated product development lead times, faster R&D feedback loops between planning, evaluation, and iteration, and overall greater control over the designability of new components, materials, and products. Having a data-driven material discovery and optimization workflow in place will ultimately allow for quicker response time to changes in the upstream and downstream supply chain.
Richards, Covestro: There are nearly countless ways the coatings value chain can benefit from AI and ML, but here is one example—the end-to-end supply chain, from the purchase of raw materials through use. There is an opportunity to leverage technology to provide greater insight and make the process more efficient through automatic tracking. In the consumer world, when you order an item online you can track it until it arrives at your doorstep. Of course, there are some challenges unique to the purchase of chemicals and coatings, and it must be done safely and compliantly, but there is an opportunity to make strides in this area. And, in doing so, we’ll open the door to utilizing that information to plan ahead and communicate with the supply chain about the resources that are needed each step of the way, so that the entire process is as safe and efficient as possible.
Kettlehorn, Dow Coating Materials: Dow makes approximately 7,000 shipments per day globally and has the largest privately owned railcar fleet in North America. This year, Dow implemented real-time tracking and delivery predictions for customer shipments through FourKites. The technology uses real-time data from the largest GPS, ELD, and telematics network and combines that with predictive analytics to provide arrival estimations. In today’s world, customers expect real time information and the ability to track their shipments. This solution allows us to focus on exceptions rather than monitor every load, set up customized alerts, or respond quickly to inquiries.
Hahn, Evonik Industries: We have always worked in close cooperation with our customers and suppliers. Now, we also want to work together on the development of digital offers, services, and solutions at an early stage. In other words, the intelligent use of data also implies meaningful interactions along the entire value chain.
Millman, Dow Coating Materials: The emulsion network generates large amounts of discrete and continuous data during batch operations. The Data Cube project in our plants gathers process inputs into a central database to compare current production with historic trends, which enables our engineers to perform troubleshooting faster and more effectively.
Ramsden, AkzoNobel: AI and ML will be instrumental in helping to find trends in data that have yet to be discovered. These techniques can help in predictive maintenance, planning, and manufacture, and in the implementation of Lean manufacturing processes not yet discovered.
The crucial element of ML and AI is that it will assist our industry in what we already do well. Strictly speaking, AI means Artificial Intelligence, but a better abbreviation would be Augmented Intelligence. These statistical techniques will work alongside us to help us make decisions with more insight, not remove decisions from us.
Formulation programs may eventually follow the automotive industry in carrying out all early stage work in-silico (as computer simulations); however, this approach is simply a way to assist in narrowing down formulation options and minimize cost, rather than allowing AI systems to design coatings for us.
Customer service will likely be an early adopter of AI-like technology. For example, Chat-Bots are already employed in other industries to provide information and guidance in an easy and simple manner to customers. Again, this type of solution does not remove the human need; it refocuses human effort on the subtle, complex, and difficult questions that need answering.
What are the biggest challenges companies in the coatings industry face when attempting to leverage AI, ML, and Big Data?
Risser, Battelle: Data quality and quantity are probably going to be big challenges. Many companies may have data sets with one to two hundred data points for trial formulations. That doesn’t qualify as Big Data and is really insufficient for effective use of ML and AI, which typically relay thousands or millions of data points. There is a need to develop intermediate solutions that enable leveraging of limited data.
A separate issue is the misapplication of these advanced tools in the hands of novices that lack proper training. It is still an issue today with these technologies that many people do not yet really understand how to use them properly. Misapplication generally leads to erroneous results, which then raises questions about the efficacy of these types of projects.
Schuetter, Battelle: Getting those millions of data points in coatings and the broader materials industry is difficult to do, largely because companies need to protect their proprietary product data and are, therefore, unwilling in general to share this type of information. These issues can generally be avoided if, for example, there is an instrument producing a stream of data regarding a specific aspect of a material of interest, perhaps a sensor providing a continuous stream of data during manufacturing or formulation. But trying to solve problems using independent, orthogonal data points will be challenging if data from many different sources cannot be aggregated.
On the flip side, there is also the potential of having lots of data but not the right types of data for a given problem. For many AI/ML models, data inputs are being used that have never been relied upon before. These inputs must be selected very carefully, because if the data is not relevant to the problem, then it won’t enable the development of effective models.
In addition, models are only as good as the data and assumptions used to define them. Regardless of the quality or scale of data used to train a model, if the assumptions built into the model are not valid, then the model will provide misleading results and have potentially unstable behavior.
Ramsden, AkzoNobel: We see three major challenges in the coatings industry to implementation of AI/ML solutions leveraging Big Data:
- Upskilling the existing workforce
- Creating and attracting new data talent to our industry
- The availability of easy-to-use tools to exploit data
Upskilling our workforce is where large and rapid gains in data utilization can take place. Our industry has a wealth of knowledge, experience, and skill—both in a technical sense and a business in general. Upskilling our existing workforce and employing these data techniques and tools in a way that augments or assists our leaders and experts is how we make data work for us.
Institutes like the UK’s National Innovation Centre for Data have a mandate to upskill workforces by carrying out subsidized projects alongside training and expert assistance.
There is also currently a lack of new talent in AI, ML, and Big Data entering the workforce in our industry, partially because these skills are relatively new, but also because advanced practitioners in these skills are rapidly employed at high wages by tech giants. The explosion in new university courses, as well as digital and data apprenticeships will, in time, address the shortage of new talent.
In the last few years, there have been many more tools available to help clean, analyze, and model data without the need for traditional coding or data skills. This advancement and data tool democratization can be considered as analogous to the advent of Windows in the early 1990s. Windows allowed the non-computer savvy to interact with PCs as tools to help carry out tasks. The new wave of data tools does exactly the same thing. Data tools such as Alteryx, RapidMiner, and Microsoft Azure ML are all targeted towards helping everyone to use data science, Big Data, and AI/ML to achieve tasks more efficiently.
Richards, Covestro: We can’t look narrowly at Big Data—it needs to be integrated throughout the organization, and this approach requires a change in mind set. Big Data isn’t a buzzword that pertains just to IT, it has implications for everyone. One of the biggest hurdles to accomplishing this change is to broadly build the skill sets needed. We believe cross-collaboration is a smart way to leverage the knowledge of our existing experts while at the same time helping others develop new skills. Doing so also requires a new way of thinking about our jobs. For example, we have a group of employees working toward degrees in data management, and they are interested in learning how we can best leverage R-squared to depict data in an easier way. With that in mind, we have encouraged knowledge-sharing by creating a team composed of people with different functional roles and from diverse areas of our business unit. Forming such teams is just one approach we’re taking to get people throughout the organization up to speed on the latest technologies.
Another challenge is avoiding data overload … efficiently parsing through the sheer volume of information we have in order to find the data that is critical to the task at hand. It’s a very real challenge and if not addressed, can be counterproductive, limiting our ability to be more nimble and better informed.
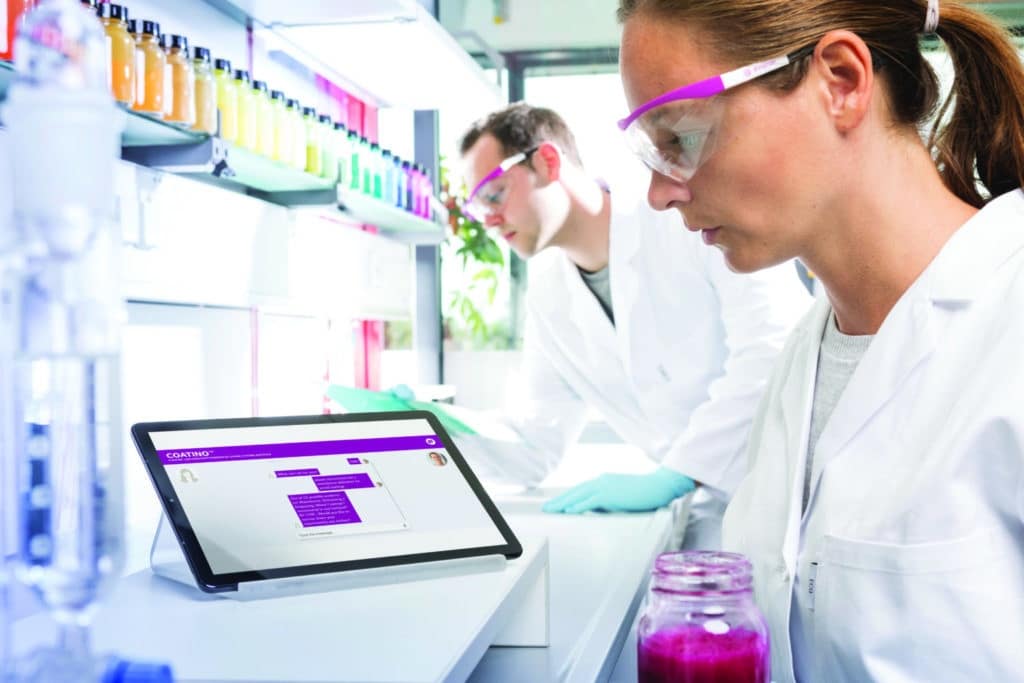
Using modern methods from the fields of Big Data science and machine learning, COATINO™ recommends the most
suitable additive from a wide range of options.
Kraus, Dow Coating Materials: Successfully implementing and adopting artificial intelligence, machine learning, and Big Data requires new organizational capabilities and a significant culture change—it is a true transformation. Fortunately, initial concerns over job losses seem to be waning, and there is a new perspective that AI, ML, and Big Data will make everyone’s jobs more interesting.
Hahn, Evonik Industries: As always, it is not about a single grand challenge, but more the sum of small challenges: completeness, validity, consistency, timeliness, and accuracy of data, among others. The often-quoted lack of skills can be overcome by adequate training and partnering with dedicated service providers. In this context, IP concerns have to be taken into account. A clear distinction of the specific domain knowledge (coatings versus algorithms) could help to address those. And last but not least, attempting to leverage AI, ML, and Big Data is a matter of change management, too.
Sapper, CalPoly: Oftentimes, early AI and ML projects must be bootstrapped alongside traditional experimental and product development workflows. This need creates an added financial cost to the project, but also tends to make the project highly visible within an organization. The high level of scrutiny may not be appropriate given the amount of risk and learning involved and also stretches personnel thinly across both the experimentational and analytical sides of a project.
In addition, there is often a challenge organizationally in creating machine learning or data-driven workflows, which largely involves changing the culture around keeping experimental data. Chemists must properly document and record all experimental data, even for poorly performing materials, so that models can learn and evolve alongside the traditional empirical experimentation cycle.
Regarding implementation, there is a real need at each organization for documented early wins in applying these tools, so that management and product development teams buy into the methods above and beyond what the popular science hype cycle might suggest.
Across our industry as a whole, there is a real need to identify pre-competitive research opportunities for industry to work on together, such as the development and publication of standard coating formulation sets, standard resin systems, and standard service life data. Each data set must include fully characterized material information in order to be useful. Trade names and qualitative descriptors like “2K epoxy” or “PDMS” will work for many models that can use categorical inputs, but highly granular structural chemical data is needed to enact any widespread change in model development and adoption across the industry.
Largely, these challenges are being overcome thanks to the intrinsic motivation of researchers, managers, and directors who have felt inefficiencies in their own material discovery processes. Despite the hype around the tools, successful implementation seems to be a matter of grassroots campaigning, always targeted at specific material or formulation challenges. Data science practitioners at chemical companies are discovering their own best ways to work together, whether that means contributing to open source programming communities like R (a statistical programming language) or Python (a general programming language with many well-developed AI, ML, and Big Data tools). They are establishing working groups or communities of practice within their own organizations. Many of these early adopters realize that their own companies have a wealth of historical data available, but it is not properly prepared for data analysis. Data extraction from dusty legacy reports is a good place to begin if you have a problem in mind and need relevant historical data.
Companies need to take a brave and bold approach to incorporating AI and ML practices within their current R&D portfolio. Don’t just hire data scientists or statisticians (although those people are key, as well!), but also encourage your scientists, chemists, formulators, and engineers to begin getting comfortable around data-driven workflows. It’s essentially a report-out culture, but one that reports data, all data, good and bad, throughout the year, as opposed to haphazard textual project reports delivered at the end of the calendar year.
Can you give some examples of how AI, ML, and Big Data are currently being used in the coatings industry?
Weernink, Dow Coating Materials: We are exploring all avenues from developing our enterprise capabilities and building the necessary infrastructure to focusing on customer insights and exploring external partners. AI, ML, and Big Data plans are embedded in our business strategy, and our leadership is embracing and driving change within the organization. We have also built centers of digital excellence that utilize AI, ML, and Big data across all functions, such as our operations, marketing, and the supply chain. In addition, we have created an active Digital Scientist network within the company to share learnings and best practices.
Ramsden, AkzoNobel: At AkzoNobel, we’ve been making paints and coatings since 1792—and we just haven’t stopped pushing the boundaries of technology. Our passion for paint and innovation has always been part of our story. That’s why our vision takes the form of one simple word—beyond—which represents both a challenge and a promise. We believe in taking innovation beyond expectation and imagination.
To make a serious and long-lasting impact, AkzoNobel is embracing AI, ML, and Big Data in many places across the company. Organization of elements such as Customer Experience, Manufacturing Excellence, and Disruption, and their continued communication is key. However, more crucial is the focus on customer value. Using tools like the Value Proposition Canvas and Business Model Canvas, we focus our data strategy on having an impact by relieving customer pains and building longer-term adoption of data techniques.
We use a system of management balancing Technology Readiness Level and Business Readiness Level in order to find external partners (both customers and suppliers) and ensure that business teams and data science teams are executing projects together.
AkzoNobel is also invested in upskilling our internal experts in new data science techniques and is well connected with partners, such as the UK’s National Innovation Centre for Data, who can help us make the most of new data techniques in our daily business.
As one example, AkzoNobel’s Paint the Future (PTF) Accelerator program was designed to engage with new technology companies, start-ups, and disrupters in the coatings industry to find partners that share our values and have exciting technology. A particular focus of the Predictable Performance initiative was to find companies that can generate supply or model new sources of data to help solve some of our customers’ problems. There are now many companies from PTF that we are partnering with, including sensor companies, robotic inspection and application companies, and additive designers.
Richards, Covestro: Leveraging AI, ML, and Big Data is being discussed at the highest level of the organization, and the focus is not just product- or IT-related. It permeates all levels of our organization. In fact, I’d say it’s becoming like safety for us. Safety is integral to everything we do, and the strategy and effort we’re putting behind digitalization is gaining that same kind of traction. It’s just that important. As such, we are collaborating with organizations to ensure that we are able to leverage these technologies where it makes sense. As an example, we are working with a university professor who is focused on ML-related tools that can be used to develop new polymers.
Hahn, Evonik Industries: Our strategy is also our mission: we want to break down data thinking and data-based acting to the level of all strategic business units and functional units and even more importantly, take all employees along with us in doing so. In fact, acting digital also means entering into new collaborations and partnerships, for example with technology companies such as IBM or Alibaba, and also with young digital companies and startups via our investment in the Digital Growth Fund I managed by the growth investor Digital+ Partners. We have also established a dedicated Cognitive Solutions team within Evonik Digital to tackle chances and challenges presented by AI and ML.
A recent example of connecting people, data, and processes is COATINO™—a voice-controlled, multi-channel platform enabling Evonik Coating Additives customers fast and easy access to innovative and sustainable solutions. This digital laboratory assistant is designed to reduce labor-intensive searches in the coatings industry. Evonik has equipped COATINO with technical knowledge and specialized expertise, enabling it to provide impressive, expert responses to even complicated questions in the area of coatings. The prototype was developed in close collaboration with European partners, and we aim to make the digital lab assistant available to the industry as early as 2020.
Risser, Battelle: At Battelle, we view the use of Big Data, AI, and ML as tools that we should be incorporating into programs whenever possible and where it makes sense to do so. In many cases, the main goal of these projects is to help our scientists free up their valuable time for novel thinking and data analysis by removing the need for them to complete rote and repetitive tasks.
It is also important to note, too, that the concepts of Big Data, AI, and ML are very broad and include a whole range of different tools and methods. In many cases, people probably don’t even realize they are already using some of these tools, but they simply don’t think of them in this context.
Schuetter, Battelle: Because we do consulting work for a variety of clients, we are focused on tailoring the application of these tools to each problem or in some cases sets of problems geared toward similar clients.
Sapper, Cal Poly: At Cal Poly, we are building natural language processing tools that can read and extract chemical information form textual documents, including patents, scientific journal articles, and technical data sheets available online. We are also developing evolutionary algorithms that can automatically discover novel polymers and coatings formulations given a desired set of material properties. We are building integration tools so that these new models may be appropriately coupled to new modes of experimentation, especially in the areas of boundaryless design spaces, flow polymerization, and autonomous synthesis and formulation. These tools will ultimately enable quicker design and discovery of materials with high performance characteristics.
Where do you see the use of AI, ML, and Big Data in the coatings industry headed over the next five years? Longer term?
Richards, Covestro: More and more, the coatings industry will come to the realization that the B2B digital experience does not exist in a vacuum. For our customers and partners, the whole mindset has changed and it is being driven by the B2C experience we have come to expect when we interact with consumer brands. In other words, we expect a swift response and a seamless experience. The chemical and coatings industries must work toward this expectation.
I think it’s safe to say that as much as we’re already seeing the impact of AI, ML, and Big Data, in the future it will transform the coatings industry in ways we just can’t predict today. As such, it’s more important than ever for organizations across the value chain to keep up with new developments, always looking for ways to push the boundaries of how we can leverage technology to advance our industry and better-serve customers.
Hahn, Evonik Industries: Currently tech companies are dominating the data-based businesses – often with a clear focus on end consumer data. When it comes to the chemicals industry in general and the coatings industry in particular. I have the impression that fixing the basics, i.e., making data fit for its intended use, is more the topic we are struggling with today. I am optimistic, though, that we will resolve the underlying challenge of data quality over the next five years. Doing so will foster the emergence and implementation of digital innovations like our COATINO digital lab assistant.
Ramsden, AkzoNobel: In the near future, the coatings industry will further embrace Big Data and digital transformation. Data will form the cornerstone of how products are developed, manufactured, and sold. This development isn’t a threat to our industry; rather, it is an opportunity to use data to get even better at doing what we do.
In the next five years, Big Data, ML, and AI will be assisting us to do our jobs more efficiently than ever before, automating some tasks, and allowing us to focus on tackling larger technical and business problems.
Robotization will continue to impact the manufacture, application, and inspection of coatings, radically reducing the amount of people exposed to dangerous environments, and increasingly allowing us to implement more Lean manufacturing efficiencies.
Personalization of business models will also begin to make in-roads in our industry. New business models will be offered to customers and suppliers through bespoke agreements, smart contracts, and service-based business models.
In addition, the coating industry will become more central in asset management. In the case of industrial assets, coatings performance will allow for longer in-service deployment, and as such, these assets will require longer-term performance passports as ownership changes.

With a fully automated paint formulation testing system, the time to find an optimum formulation is reduced.
Over 100 samples can be tested within 24 hours on this Evonik system.
For formulators, greater transparency of conditions in-field from sensors and environmental data modeling will become widespread. Maintenance of coated surfaces will become more bespoke as these surfaces are monitored and modeled. The way products are guaranteed will change—new business models around data-driven maintenance cycles will become more prevalent and are already being embedded in some industrial applications.
Overall, technology and data science will become more prevalent globally, and the industries that embrace AI, ML, and Big Data as tools to help manufacturing, satisfy customers, and create new business models, will flourish.
Eckersley, Dow Coating Materials: Heritage material companies will continue to address the challenge of transforming the way work is done. In many cases, they have already built expert systems and now have to transition them to incorporate AI, ML, and Big Data. Companies will also be building new employee skillsets; today, everyone needs composite skills—their fundamental work combined with the ability to work with Big Data, AI, and ML. In other words, marketing is transitioning to Digital Marketing and R&D is transitioning to Digital R&D. That is because at their core, AI, ML, and Big data are helping people do work better and more quickly, reduce non-value added, repetitive tasks, and free up time for more value-added activities.
The advent of Big Data, AI, and ML represents the 4th Industrial Revolution. With these new capabilities, we will be able to find new solutions to unsolved problems and create a better world. It will be a journey that if done right will create exciting new careers, explosive innovation, and effortless and enjoyable customer experiences.
Sapper, Cal Poly: We are in early days as far as machine learning adoption in our industry goes. We are just coming to terms with the necessity of taking statistical, designed experiment approaches to synthesis and formulation. Similarly, the ideas of combinatorial synthesis, high-throughput experimentation, and roboticized materials science are just now becoming commonplace within certain organizations. As we get used to these methods, we will naturally begin to discover deficiencies in them, including design space constraints and the problem of material enumeration, coupled with a large and fast influx of experimental data that will be a new challenge to many researchers and teams. When this happens, data will become too large to easily handle using the traditional tools of paper notebooks, spreadsheets, and fitted trendline models. At the same time, material requirements will become more demanding, along with shorter expected development and turnaround times. Material interactions in complex formulation systems will be too complex to model using previous, regression-based approaches. At this point, researchers will understand that data-centric approaches to material generation, experimentation, and exploration are needed.
It will be slow going at first, as these methods must run in parallel with existing experimental approaches. Ultimately, though, within the next decade, I would say, the data workflows and the ability to design or propose new materials and formulations with a high degree of fidelity in virtual settings will surpass our ability to quickly discover these formulations in the lab using pure empirical methods. At this point, lab experimentation will be highly automated, and much of the day-to-day synthesis and formulation work will fall in the domain of experimental design and model building, by data scientists, chemists, and formulators.
Ultimately, the impact of artificial intelligence and machine learning in our industry will be large and unavoidable. The job of a bench chemist will disappear in our lifetimes, replaced by automation and robotics. This does not mean we will not need trained chemists and formulators, but that their job descriptions will change. Technology and material needs will evolve faster than they do now. Planning automated experiments, analyzing data, building and deploying predictive models, and evolving workable new formulations from data and derived models will be the job of the coatings formulator in the future.
CoatingsTech | Vol. 16, No. 9 | September 2019